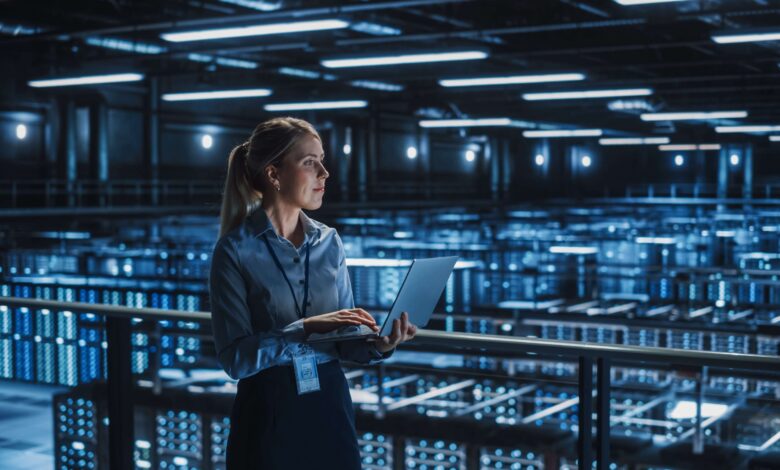
Artificial Intelligence (AI) is the biggest technology inflection point since the rise of the internet, and its ongoing impact on global industries cannot be overstated. When it comes to IT operations and infrastructure, emerging AI technologies have already saved thousands of global enterprises significant time and money through automation and insight while simultaneously assuring optimal end-user experiences. And this is only the beginning.
By extending AI from the end user across all layers within a network environment, I believe that network outages, trouble tickets, and application downtime will eventually be a thing of the past. Yet, for AI-powered networking to be truly optimized, it must be embedded in every step of the user journey with solutions designed from the onset to leverage the right data via the right infrastructure to deliver the right real-time outcomes. This is what we call AI-Native Networking.
An AI-Native Networking Platform has two primary use cases. Most prevalent is AI for networking, where AI is used for IT operations (a concept known as AIOps) to identify patterns, predict network behavior, detect anomalies, and make proactive suggestions or self-driving corrections to optimize user experiences. This ensures that every connection within a networking environment is reliable, measurable, and secure for every device, user, application, and asset used by the end user.
The second is networking for AI, a massive market that is emerging whereby data center networks are essential to support stringent requirements for AI training and inference workloads. These environments require extremely fast and reliable GPU interconnect, low power consumption, and agility, making the network critical to the ongoing success of AI initiatives.
AI for Networking: Assuring the Best User and Operator Experiences
IT professionals and organizations of any size can all benefit from AI-Native features that derive simpler, more productive, and more assured experiences at scale. This results in benefits like reduced configuration errors, streamlined and automated workflows, and the delivery of reliable, secure, and high-quality user connections.
Deploying and scaling new networks brings a lot of complexities, with human error often driving the bulk of configuration issues. An AI-Native Networking Platform allows operators to proactively identify and resolve issues, thus reaching a level of efficiency that allows them to devote their attention to innovating digital services and applications for the end user.
For businesses to scale, they need to manage increased traffic with the same or reduced budgets and a smaller workforce. To accomplish more with fewer resources, operational teams need to elevate from routine tasks to strategic thinking, which will allow them to concentrate on driving business results. AI-Native Networking allows operators to implement insights without the need for human intervention—often before any problems result in possible downtime or poor user experiences.
The third benefit is the delivery of reliable, measurable, secure, and high-quality connections for each user, even as the workload demands increase. Users need to have confidence that, regardless of the scale and complexity, connectivity will operate seamlessly and securely, reduce human error, and provide an optimized experience. AI-Native Networking minimizes the risk of network unreliability down to the device, user, application, and session level.
Networking for AI: Connecting the AI Revolution
As data center footprints and compute requirements continue to significantly scale within and across regions, the role of networking becomes increasingly critical. This is particularly true for handling fast-growing and performance-intensive distributed AI workloads, such as LLM training and distributed inferencing.
Modern AI/ML clusters comprise hundreds or sometimes thousands of GPUs in a data center that provide the massive, parallel computational power that is required to train today’s AI models. Distributing workloads across GPUs and then syncing them to train the AI model requires a new type of network that can accelerate job completion time (JCT) and reduce the time that the system is waiting for that last GPU to finish its calculations (tail latency).
AI-Native Networking makes it possible to more effectively build and reap the benefits of AI applications while saving substantial time and money.
Enhanced Experiences with Virtual Network Assistants (VNAs)
Whether doing AI for networking or networking for AI, both environments can benefit from VNAs. VNAs enhance the network’s ability to auto-generate solutions, predict potential issues and automatically configure or optimize the network’s performance. They deliver this through a conversational interface that leverages natural language processing (NLP) and natural language understanding (NLU), so complex problems can be handled via simple language queries. Recently, generative AI (GenAI) has entered the equation to help improve a VNA’s capabilities.
These VNAs function like networking specialists, and they’re designed to support network professionals, including network administrators, site reliability engineers (SREs), or anyone based in network operations centers (NOCs). Functioning in this way offers various business benefits, including ease of use and scalability, providing real-time responses, task automation and workload reduction, and more.
There are even VNAs available that incorporate conversational AI into networking operations, revolutionizing how IT departments communicate and interact with enterprise networks. By continuously learning from the data, applications, and network devices provided, the virtual assistants are equipped with a wide range of knowledge and a high level of efficiency. From this, more challenges can be resolved even faster and with enhanced accuracy. As a result, VNAs can maximize user and operator experiences while reducing IT costs.
One key capability of a VNA is the ability to automate troubleshooting, which can quickly identify and diagnose network issues and decrease workloads related to network problem-solving. Another key aspect is proactive network management. This actively monitors the network environment needed to preemptively mitigate potential disruptions, ranging from misconfigurations to security weak points.
Additionally, the use of a VNA enables the network to learn from patterns, adapt to new conditions, and make decisions effectively. This reduces the risk of human error and the need for human intervention, essentially transforming the network into a proactive, self-healing, and self-optimizing entity. This ultimately improves reliability, security, and—most importantly—the user experience.
Unlocking New Advancements with AI-Native Networking
With an AI-Native Networking solution in place, organizations can proactively determine the details of a network performance issue long before it can impact the user experience. This makes it a game changer for IT and network operations. The technology can also successfully transition organizations and IT professionals from reactive to proactive troubleshooting—and even enable predictive network troubleshooting and management. This is particularly important for organizations within critical national infrastructure (CNI), such as healthcare, government, and education. Within these organizations, networking services are essential to support internal and external communication, enhance and advance operations, and improve the lives of end users, customers, and the general public.
Ultimately, the advent of AI-Native Networking and the integration of VNAs marks a transformative period in global digital connectivity—achieved by extending AIOps capabilities across all facets of network operations. From end-user devices to application infrastructures, AI-Native Networking offers a promising solution to recurrent challenges in maintaining network performance. This includes the ability to mitigate network outages, expedite troubleshooting, and greatly minimize application downtime.
The benefits of AI-Native Networking offer unprecedented levels of automation, intelligence, and reliability. By leveraging AI algorithms to automate tasks and analyze vast volumes of data to predict network behavior in real-time, AI-Native Networking promises to elevate the current state of network connectivity. With it, organizations are positioned to unlock new business potential and drive the evolution of networking into the next era of innovation and efficiency.