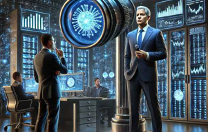
Analyzing corporate earnings calls has long been central to investment decision-making. These calls offer important live opportunities for executives to share previously material non-public information that can shape market perceptions of a company’s outlook, strategy, and risk profile. Yet as crucial as these discussions are, the volume and complexity of data presented can make it difficult to parse in real time. Executives are well aware that these calls can send signals to the market and are increasingly adept at controlling their narrative.
AI-driven investment analysis tools are reshaping how investors process earnings calls. AI-powered speech analytics, NLP (Natural Language Processing), sentiment analysis, and vocal forensics now offer investors deeper insight into what’s truly being said, and sometimes, what’s conspicuously left unsaid. As has been the case in the age-old cat and mouse game between management and investors, these emerging methods have prompted C-suite executives to evolve their disclosure strategies. This interplay of technology and human communication is changing the dynamic of earnings calls in ways both subtle and profound.
The Evolution of Earnings Call Analysis
For decades investors have studied corporate communications to detect changes in tone, gauge optimism or caution, and spot red flags that could foreshadow risk events. Traditionally, this work was done manually or through basic keyword searches, focusing on how often certain terms like “headwinds,” “guidance,” or “uncertain” appeared in a transcript. While helpful, these methods offered limited insight into the deeper subtext of management’s statements.
Today’s AI-driven approaches go far beyond simple word counts:
- Text-based NLP identifies sentiment, urgency, and possible evasiveness (weak modal words) in real time by examining language structures, synonyms, and context.
- Voice analytics detects variations in pitch often inaudible to the human ear, pace, and vocal stress, providing clues about underlying emotional states such as confidence or anxiety.
- Topic modeling algorithms track how thoroughly management addresses core questions, or how consistently executives discuss planned initiatives over multiple earnings cycles.
Once relegated to sophisticated hedge funds or academic research, these tools are becoming mainstream, transforming investor understanding.
AI-Enhanced Methods for Parsing Disclosures
- Predictive Insights:
Beyond analyzing what was said, AI predicts how changes in management tone or discussion topics correlate with future stock price performance. Large commercial data sets are now available to hedge funds and empirical investors to fine tune their voice-based trading strategies. - Natural Language Processing for Nuance
Modern NLP algorithms do more than just label statements as positive or negative. They interpret context, measure shifts in rhetorical complexity, and highlight unusual jargon or buzzwords that might signal something the company is trying to emphasize, or avoid. Investors can compare current calls to historical calls to detect changes in the executives’ diction or tone. If, for instance, a CFO switches from a confident “we see strong long-term demand” to a hesitant “it remains to be seen,” NLP tools can immediately flag that shift for investor attention. - Advanced Sentiment Analysis
Basic sentiment analysis often lumps words into “positive,” “negative,” or “neutral.” New AI-driven techniques incorporate sarcasm detection, domain-specific language training, and context-specific weighting of terms. For instance, an executive’s statement about “strong operational challenges” might not necessarily be purely negative if the subsequent discussion reveals the company is handling these hurdles effectively. The contextual layer of AI solutions can place words, phrases, and even entire Q&A sections into a more holistic framework.
- Vocal and Behavioral Analytics
Voice-analytic methods measure subtle markers in real-time audio feeds. Algorithms can detect:
- Pitch fluctuations and Voice Tremors that may indicate stress or uncertainty.
- Speech rate changes when executives accelerate through sensitive topics.
- Hesitation patterns such as frequent filler words (ie. Ah or Um), stutters, or elongated pauses and even throat clears.
Speech is physiological and reveals emotional states beyond the content of words. An abnormally high pitch might indicate excitement (or anxiety), while slower, more deliberate speech accompanied by vocal tremors could signal caution or an attempt at obfuscation. These new dimensions of vocal data, complementing textual transcripts, give investors a more robust understanding of the information being conveyed.
- Executive Q&A Focused Analysis
As most prepared remarks are now pre-recorded, the analysis tends to focus on the unscripted Q&A section on the call. AI models now rank how thoroughly management answers analyst questions. By comparing the semantic similarity of questions to the responses, investors gauge whether executives are staying on-topic. If a CEO or CFO repeatedly pivots away from the question asked, advanced language models can highlight those evasive patterns. This helps identify the possibility of withheld information or unwarranted optimism. Investors value direct, consistent communication, so metrics that measure how “on-topic” an answer can be especially revealing.
Management Is Responding
Executives themselves are not operating in a vacuum; they are highly aware that AI is listening. Prepared remarks and Q&A strategies increasingly account for sophisticated machine-driven parsing. Common responses to these technologies include:
- Tighter Messaging and Vocabulary Control
Many executives stick to carefully vetted language, aiming to minimize unintentional signals. Teams rehearse wording that will sound consistent and confident. Certain negative words might be replaced with softer alternatives to avoid triggering negative sentiment analysis. For instance, “we encountered operational failures” might become “we navigated operational complexities.” - Strategic Tone Management
With the assistance of their Investor Relations professionals, senior management frequently train with voice coaches or communications experts to regulate pitch modulation and pacing, knowing that dramatic fluctuations can catch the attention of AI-driven emotional analytics. The goal is to project steady confidence even if the underlying fundamentals are volatile. - Pre-emptive Disclosures
Some companies proactively address topics that might come up in Q&A before analysts ask. This is sometimes referred to as “preempting the pivot.” By providing structured narratives in prepared remarks, executives attempt to shape the conversation, limiting potential follow-up questions that can veer into uncomfortable territories. - Coached Evasiveness
While direct honesty remains the gold standard for building market trust, there are scenarios where executive teams have reasons to remain vague, such as ongoing negotiations or compliance constraints. Coached evasiveness can entail using broad, forward-looking statements: “We are evaluating multiple options to drive long-term growth,” thereby providing minimal fodder for AI sentiment detection.
The Impact on Investors and Markets
- Faster Reaction Times
AI-driven analysis of earnings calls can run in near-real time, flagging unusual changes within minutes. This immediacy can produce quicker shifts in stock prices as traders act on fresh data. While this can boost market efficiency, it also raises volatility risk if the data’s interpretation is imperfect or if rumors spread before thorough verification.
- Democratization of Research
Previously, only large institutional investors had the resources to maintain big teams of human analysts listening to calls in real time. Today, smaller funds and even individual investors can access AI-based transcripts, sentiment dashboards, and voice analytics. This levels the playing field, though it also intensifies competition in generating alpha.
- Emphasis on Transparency
The more advanced the AI becomes; the less room executives have for obfuscation without being caught. In the long run, this trend may encourage better corporate behavior and more candid communication with shareholders. Over time, capital is likely to flow toward companies that cultivate trust by providing consistent, transparent statements.
Future Trends
The technology behind AI-driven investment analysis evolves rapidly. Some imminent developments include:
- Multimodal AI Systems: Combined text, voice, and even facial expression analysis (for video-based calls) to build a complete emotional and contextual map of the speaker’s psyche.
- Language-Model Powered Q&A: Real-time generation of clarifying questions that could push management to explain ambiguous points on the spot.
On the corporate side, we expect to see:
- AI-Informed Pre-Briefing: Management teams using AI internally to run mock calls, identify potential red-flag language, and refine the final script.
- Augmented Investor Relations: IR departments employing advanced analytics to craft “talking points” that keep linguistic sentiment and voice tone consistently aligned with strategic goals.
- Management use of voice cloning: One company has already used voice cloning in the prepared remarks section of their earnings call. This reduces the need for editing and re-takes and allows the introduction of a specific emotion to stress points made.
These trajectories highlight a feedback loop where AI is both an observer and a catalyst for changes in the information that gets disclosed and the way it is presented.
Conclusion
AI-driven technologies for parsing executive disclosures have accelerated the circular relationship between corporations and investors. Powerful NLP, sentiment, and voice analysis tools now make it possible to interpret nuanced cues from subtle changes in phrasing to fluctuations in vocal stress. As a result, both the content and delivery of earnings calls have become more scrutinized and carefully managed.
Executives, aware of these technological capabilities, are adjusting their communication strategies, sometimes opting for hyper-controlled messaging while at other times striving for transparency to build greater credibility. The upshot for market participants is a more data-rich environment where signals of confidence, caution, or even deception are quickly identified and priced in.
AI-driven investment analysis stands to redefine how earnings calls are conducted, how stakeholders interpret corporate narratives, and ultimately, how markets function. As technology continues to advance, the ideal scenario is one that benefits both companies and investors and will be characterized by more authentic, data-backed communications and more efficient capital allocation decisions.