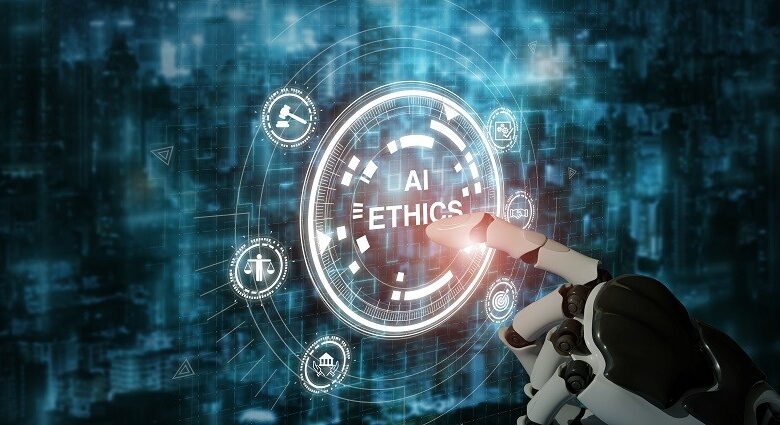
Integrating machine learning into healthcare systems brings game-changing benefits and grave ethical and legal challenges. Optimum care concerning compliance results from striking the appropriate balance between innovation and regulations.
Innovation vs Compliance
The rollout of these technologies happens by regulatory frameworks as they become necessary. Understanding the dynamic between HIPAA and AI is crucial as healthcare systems increasingly rely on machine learning to improve patient care.
Rules, called regulations that require patient data to be treated confidentially and securely, exist, and HIPAA mandates them. AI tools that protect patient information from unauthorized or breached access must fulfill these regulations.
AI systems can analyze large datasets to improve decision-making, optimize treatment plans, and enable optimized operational efficiency.
Better Diagnostics and Improved Outcomes
AI can read medical data patterns and thus notice diseases earlier and more accurately diagnose them. With machine learning algorithms, cancer can be detected very early, leading to a high possibility of curing the disease.
With these insights, healthcare providers can better prospects for patient outcomes and healthcare system strain.
Increased Operational Efficiency
The admin manually maintains routine administrative tasks like scheduling, billing, and so on so that healthcare professionals can constantly focus on patient care. Beyond that, predictive analytics improve resource allocation by predicting patient demand and operational efficiency.
Ethical Data Use
Data is the backbone of AI – it has become an essential piece of the puzzle for almost anything AI. However, collecting and using patient data without explicit consent raises ethical issues. Healthcare organizations must put data practices into view, telling the patients their information would be used, and must also gain consent.
Bias in AI Systems
Just like the data used to train them, AI systems are only as unbiased as AI systems are as unbiased as the data they are trained on. If the datasets the AI models are trained upon have historical biases, those biases can be possibly perpetuated or even further increased by the produced AI models.
For instance, AI diagnostic tools designed using an underrepresented demographic group in the data will yield less accurate results for that group. Healthcare organizations will then follow suit and adopt diverse datasets and audit their AI systems regularly to counter this problem.
Challenges in Compliance and Solutions
Several challenges exist in bringing AI technology into healthcare, with healthcare providers needing to always work within the framework of current regulations.
To remain compliant, healthcare organizations must be tuned in to these changes. It is essential to receive regular staff training and updates and communicate closely with legal and compliance teams.
Audit and Accountability
Such an AI system must have a clear audit trail so that we are accountable for its actions. Documenting every data access, modification, and use shows compliance if you are audited, and it assists you in identifying slips in the system.
Real-Time Monitoring
Running AI tools in the background to help monitor compliance in real time is a great way for companies to head off potential risks. With automatic alarming of any suspicious activity or activity out of the standard procedural protocol, healthcare providers can promptly exercise possible actions to correct any suspicious activity.
Transparency in Decision-Making
The lack of transparency is known as the “black box” problem; without explanations of how AI software arrived at a specific conclusion, healthcare providers may find this obstacle hard to figure out. To handle this, explainable AI (XAI) models explain what the model has decided to do and how it arrived at the conclusion.
Patient Consent and Autonomy
The autonomy of patients is an important theoretical principle ethically in health care. Patients should be able to use AI tools, if implemented appropriately, at their discretion, and to the extent possible, understand how and why they are using them as part of patient care and consent to it.
It means openly and honestly communicating what an AI tech can do or, even more importantly, what it can’t do.
Accountability in AI Errors
Although they can improve accuracy, AI systems make no mistakes. Finding ways to blame someone when code doesn’t work is hard. So, we first have to put in place clear protocols that our healthcare organizations will use to manage errors related to AI so that patients get the remedy they deserve and the system itself can be improved so we don’t make the same mistake again.
Conclusion
AI technology has huge potential in the healthcare domain, but ethical and compliance frameworks must ensure it is under control.
By understanding the dynamic between HIPAA and AI, healthcare organizations can leverage these innovations while safeguarding patient rights and maintaining regulatory compliance. We must find a way to address these priorities in the digital age to develop trust and provide high-quality, ethical care.
Balla