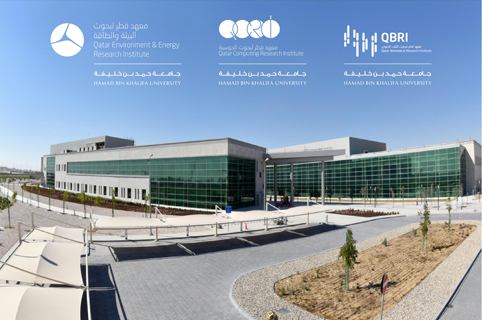
The COVID-19 pandemic demanded an urgent response that vastly accelerated typical drug discovery timeframes. The global health community had months, as opposed to years, to find a solution. Faced with this challenge, drug repurposing offered great potential in finding an effective treatment quickly.
By repurposing drugs that have already been trialled and approved, the slow processes of de-novo drug discovery – developing a new medicine from scratch – could be avoided. However, repurposing still requires extensive analysis of drug compounds, and identifying which compounds can successfully treat an illness is also a time-consuming process if done by trial and error. Considering the ever-developing variants of COVID-19, a traditional drug discovery process was one that the world could not wait for. That’s where machine learning came in.
Finding COVID-19 treatments with machine learning
Despite machine learning and Artificial Intelligence (AI) being a relatively new introduction to the world of clinical development and biotechnology, their ability to deal with large amounts of data has already proven to be highly efficient in the sector, allowing scientists to screen en mass at a rate far beyond human capability. In drug repurposing, they enable the medical community to quickly identify existing sets of drugs that can be trialled for the treatment of viruses and diseases – essentially helping us to quickly find the needle in the haystack.
At the Qatar Computing Research Institute (QCRI) – part of Qatar Foundation’s Hamad Bin Khalifa University – we have been contributing to a global field of work that is using Artificial Intelligence to uncover which compounds from existing drugs can be re-purposed to fight COVID-19. We developed a bespoke machine learning model that has now successfully identified 47 suitable drug compounds that have the potential to inhibit the COVID-19 virus, out of a database of 1,482 compounds.
An antibiotic compound named Brilacidin was proven most likely by the AI model to be able to combat the virus. From other predictive modelling studies, there is a host of data that supports that Brilacidin can effectively treat COVID-19, and the compound has now received FDA Fast Track designation for potential treatment.
The study conducted at QCRI builds on a developing body of research that highlights there is great potential for using machine learning to identify “key players” in drugs and repurpose them efficiently for the treatment of unfamiliar viruses and diseases.
Leveraged properly, it is evident machine learning can propel drug discovery forwards, and help us to tackle the medical challenges the world is currently facing and will continue to face in the future.
Integrating AI in the wider health system
AI-driven research is making positive waves in the wider healthcare community too.
Through its deep and intricate analysis of extensive data, AI can provide tangible conclusions of global medical trends to the every-day clinician. This increased accessibility to research will encourage a well-informed ecosystem of knowledge sharing amongst professionals, facilitating a more transparent and improved healthcare system. Ultimately, integrating AI in medical research will also help healthcare professionals to give better-informed diagnoses and advice to patients.
A global mentality
AI can also help medical research to become more representative.
Too often, research has been based on analysis of European and North American populations. Due to the speed in which research can be carried out through AI, however, there is an opportunity for new and vast data sets to be analysed. A new analysis of global clinical data sets will mean suitable, tailored treatments can be found for a much wider range of people, from different communities, ethical groups, and physiological builds. This will avoid the risks we run currently of generalising smaller studies to the global population and increase the inclusivity of medical research and subsequent solutions.
Viruses have often been found to have a range of effects on different populations, so improving inclusivity in medical research is essential.
Precautionary measures
Despite the numerous benefits of applying AI and machine learning to health research, both researchers and practitioners must take precautions. As the use of AI is a relatively new introduction to medical research, it can be expected that some patients and clinicians may distrust the accuracy of AI-based findings. Some might also have concerns about confidentiality. AI-based medical research relies heavily upon large amounts of patient data, and it is vitally important for datasets to be kept anonymous.
Trusted medical bodies such as the World Health Organisation are currently developing a global digital health strategy to regulate global governance of data handling in healthcare. This formal recognition of the regulation needed will hopefully be a first step towards encouraging greater trust in AI-based decisions and treatments. However, it is also the duty of researchers and practitioners to work towards improving public understanding and acceptance of AI in the sector.
Forward thinking
It is evident that the use of AI has the potential to propel medical discovery forward – and this is a much-needed accelerator. The COVID-19 pandemic demonstrated how easily the world could be caught unawares by a new and hard-hitting virus. Incorporating and trusting in AI-driven processes will greatly enrich our understanding of drug-repurposing and medical research so that we are not only in a better position to continue to tackle COVID-19, but also more prepared to tackle news viruses or diseases that the future will bring.
Brilacidin from Innovation Pharmaceuticals in one of those thought to kill Covid, created with AI some years ago. Can’t wait to hear the results on November 8, 2021