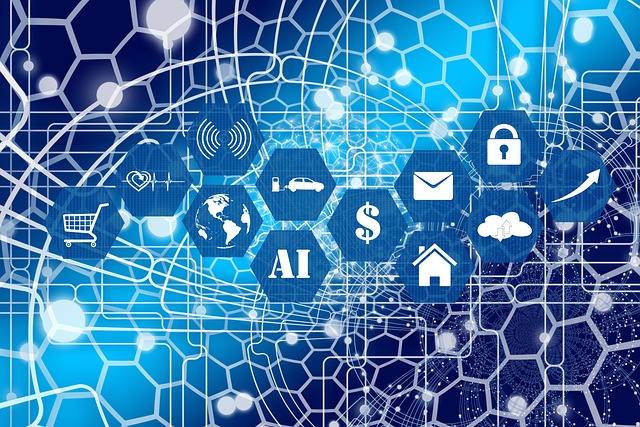
“Computers will overtake humans with AI within the next 100 years. When that happens we need to make sure the computers have goals aligned with ours” – Stephen Hawking
A recent report by the World Economic Forum predicts around 7.1 million jobs will be replaced by artificial intelligence (AI) by 2020.
Another prediction by Forrester contends that the loss would be over 9.1 million jobs by 2025.
According to CBInsights, there is a sharp increase in global investments in AI from $282 million to over $3 Billion from 2011 to 2016.
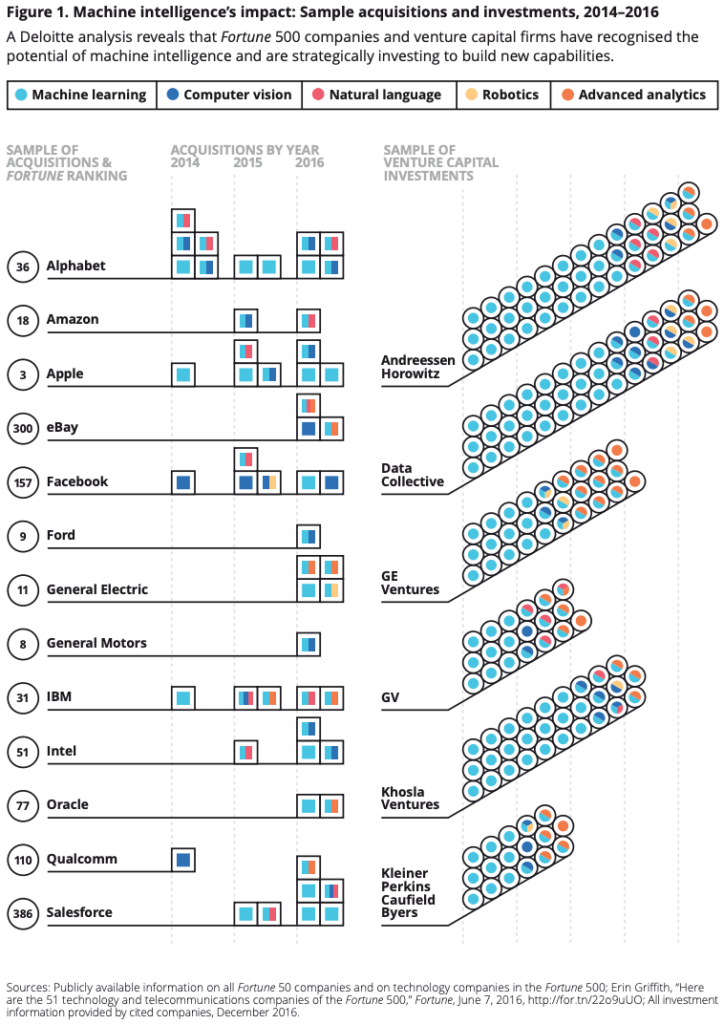
In the above graph by Deloitte, we see that all major organizations have immense investments in AI and machine learning, with Google/Alphabet leading the pack and aim to continue to do so by acquiring new companies.
Although there are a number of enterprises utilising AI and Machine Learning (ML), the good news is that AI is still in infancy and its entire capability is not tapped into.
A detailed report by McKinsey states the following:
“AI adoption outside of the tech sector is at an early, often experimental stage. Few firms have deployed it at scale. In our survey of 3,000 AI-aware C-level executives, across 10 countries and 14 sectors, only 20 percent said they currently use any AI-related technology at scale or in a core part of their businesses. Many firms say they are uncertain of the business case or return on investment. A review of more than 160 use cases shows that AI was deployed commercially in only 12 percent of cases.”
In this article, I will present the fundamentals of AI and what you need to do in order to adopt AI and machine learning in your organisation.
Understanding AI and machine learning
The most important technology with regards to AI is machine learning, a machine’s ability to perform a task without human intervention and with incremental performance improvement.
Examples range from Amazon’s recommendations for products that you might like to Facebook recognising your friend in an image.
Machine Learning is fundamentally different from how typical software code is written.
Machine learning is able to learn by using examples.
A machine is given millions of training inputs, for example, the inputs can be various images of animals: dog, cat, mouse and the outputs would be the correct labels of these images.
Once the system learns these, it utilises this information to correctly predict a new image it comes across.
Any business problem where you have a large amount of data and are trying to get an outcome is a good candidate for the application of machine learning.
Although AI is often envisioned as a humanoid-robot, this type of algorithmic AI is the most common use case in today’s world.
For example, JP Morgan introduced a new AI program that reviews commercial-loan agreements that, until the project went online in June, consumed 360,000 hours of work each year by lawyers and loan officers. This is now reduced to a few seconds.
“We’re willing to invest to stay ahead of the curve, even if in the final analysis some of that money will go to product or service that wasn’t needed,” Marianne Lake, JP Morgan’s finance chief, told a conference audience in June. That’s “because we can’t wait to know what the outcome, the endgame, really looks like, because the environment is moving so fast.”
AI and machine learning implementation strategy
Everyone understands Apple’s Siri, Microsoft’s Cortana, Google’s OK Google, and Amazon’s Echo.
What we don’t understand is how we can utilise AI in our enterprises.
We grapple with questions like:
- What are my options to pick the right machine learning technology?
- How can I make AI and machine learning technology an investment that produces good returns?
- When do I act to get ahead of competition?
While these questions are valid, they form the proverbial “putting the cart in front of the horse”.
Instead think about:
- A business problem that needs to be solved that impacts the top or bottom line
- What would the business process transformation look like?
- Can emerging technologies like Machine Learning and AI solve my problem quickly and reliably?
In the same report by McKinsey, we notice 6 important characteristics of AI adoption.
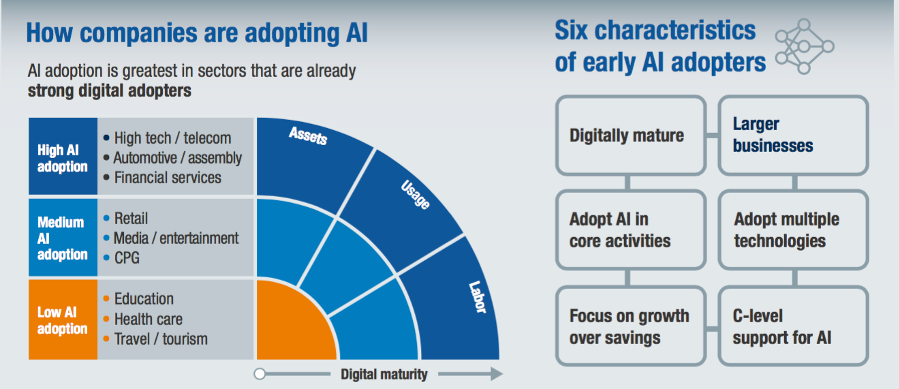
Successful implementation factors
Studies and reports from various sources suggest the top three success factors of AI implementations:
- Open organization culture and change management
- Business use cases
- Data ecosystems and digital readiness
Organization Culture: Research reveals that leaders who use emerging technologies to create transformational business models drive successful AI adoption.
They have a high sense of urgency and break the silos in their organization by an organization-wide adoption strategy. They empower the employees to learn and deliver these innovative solutions.
It is also important to understand that bolting AI and machine learning solutions on top of legacy applications is detrimental to overall business goals.
Having your digital platforms ready, adopting a digital transformation mindset and setting up easy integration points to various systems and applications would ensure your solution is robust, scalable, and worth the investment.
One Comment