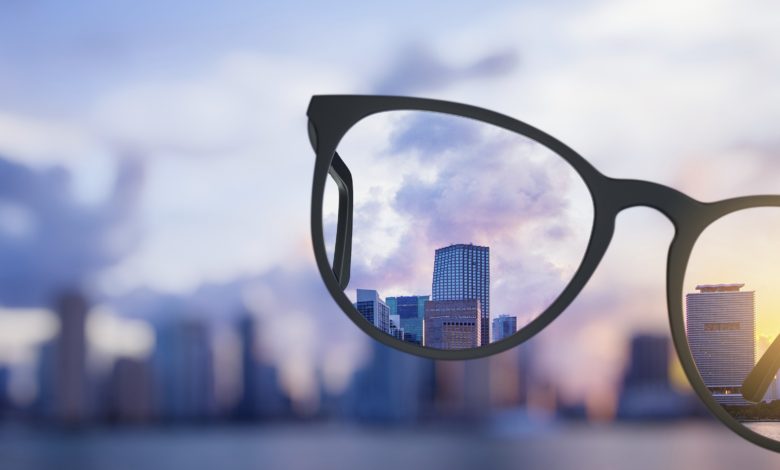
Four weeks ago, my last article for The AI Journal pointed out that the COVID-19 “creates data deficits … Relaxed or suspended regulatory reporting requirements deliver less data to governments for aggregation, which in turn delivers less complete data sets to markets.”
It did not take long before the official sector started pointing out crucial breaks in key data sets used for global macro and credit risk analysis.
Missing Inflation Data
De Nederlandsche Bank (the Dutch central bank) recently released research showing that key inputs for HIPC inflation measures were not collected during March and April. Because the HIPC data is only updated annually, when central bankers sought to plug the gap in order to conduct monetary policy, they only alternative was to use same-month 2019 data as a proxy for 2020 prices in key sectors disrupted by the pandemic.
While the data gap was resolved, actual price data diverged significantly from the estimated data. AND the composition of consumption changed materially during the period, raising real questions about the predictive nature of HIPC data going forward. Actual inflation was much higher than either estimated amounts AND headline inflation:
It is fair to assume that the Netherlands is not alone in experiencing these discrepancies even if it might be the only central bank currently shining a spotlight on the situation.
Challenges for artificial intelligence systems
Supervised machine learning aficionados everywhere will find this development helpful for a range of reasons.
At its most basic, the situation underscores the importance of human engagement and supervision of the machine learning process that delivers artificial intelligence. These human jobs are safe from outsourcing. In fact, they have become more important overnight.
As I wrote in The AI Book last year, in a chapter on the future of work, the near future is not a man vs. machine universe. Instead, AI delivers the promise of enhancing the ability for humans to accelerate the cognition process in order to deliver new insights faster and better than in the past.
To realize this future, the key first step is to ensure that AI systems are trained on solid data.
AI systems, just like people, can only generate analysis as good as the data they are fed. Data quality may be the dull part of the AI universe, but it is crucial to get the initial training data right.
Automatically delivering economic or other traditional data to AI systems for scenario analysis purposes without quality control processes risks highly problematic outputs particularly in the AI context due to the inability to access audit trails or other mechanisms to verify how the data was used.
The Dutch central bank research illustrates that data gaps present a far from trivial challenge. The divergence between estimated and actual inflation data was large. Training AI systems to distinguish between estimated and real data requires nuance and supervised learning protocols, and that is before we start talking about factoring in ex-post data restatements.
These challenges are just the tip of the iceberg for financial firms seeking superior scenario analysis to support risk assessments regarding potential default rates and loss given default rates. Large discrepancies regarding real and estimated inflation rates were only spotted with a long lead time; in the interim, humans as well as AI systems will have generated mistaken calculations if they did not know how to read and interpolate the fine print properly.