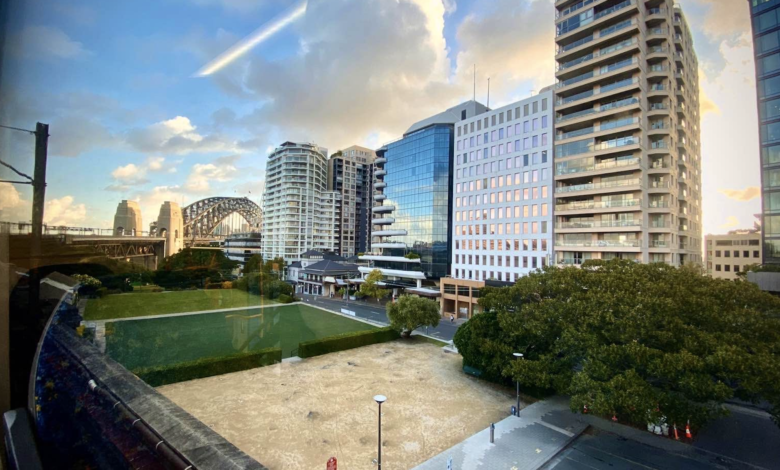
Advances in behavioural science provided a number of interesting developments for the financial sector, its service provision, as well as marketing techniques deployed within the sector.
Behavioural design interventions and nudging experimentations revealed, for example, that designing smarter default options help people save more and repay their debts more quickly; simple, salient and interactive product information improves customer comprehension; timely prompts help people save money and seek financial advice when they need it; decision-support systems (e.g., offer blockers, card controllers) help people make better financial decisions; emotionally engaging rewards and incentives make financial products and services more attractive, etc.
Yet, most recent developments in behavioural data science through the emergence of behavioural AI paved the way for a much more ambitious agenda, which is revolutionising the finance industry as a whole and FinTech sector in particular.
What Is Behavioural Data Science and Behavioural AI?
Behavioural data science refers to a new field, combining cross-disciplinary methods from decision theory, behavioural science, economics, psychology, data science, computer science, engineering, mathematics, statistics, as well as other fields to design new modelling approaches in order to better understand and predict 3 strands of behaviour: (i) human behaviour (e.g., consumer decision making); algorithmic behaviour (e.g., detecting and preventing algorithmic bias as well as understanding the interactions between algorithms and humans); and systems behaviour (e.g., designing complex but effective systems to maximise people’s potential).
While behavioural data science represents a combination of theories, models, methods, and approaches; behavioural AI is a set of applied tools, which connect behavioural data science with practice.
Why is Behavioural AI Particularly Powerful in FinTech?
Behavioural AI makes the most impact in the FinTech industry due to the nature of the sector and its extreme data-density.
Behavioural AI directly interacts with the FinTech secret sauce, allowing us to take the data-driven financial services to a completely new level. When we talk about the FinTech secret sauce, we imply major innovations and business models associated with them, which are delivered to customers in effective, transformative, and disruptive ways.
The FinTech secret sauce is inherently dynamic and fast-moving, leveraging on the most recent data, business models, and business model innovation in the banking/financial sector delivered through digital means.
So, what are the main flavours of the FinTech secret sauce?
Which has emerged in the last few years?
Several notable examples include, but are not limited to:
- Personalised and adaptive finance – provision of individual-based, flexible financial services with human-in-the-loop approach (e.g., Revolut, Monzo, etc.)
- Encrypted cryptocurrency trading – simplifying cryptocurrency trade operations (multiple providers around the globe)
- Cross-border e-commerce and payment – facilitating international commerce and payments (e.g., M-Daq)
- Global financial remittance service – catalysing global financial transfers and gift-giving (e.g., TransferMate)
- Customer identity verification and fraud prevention – managing customer digital identities (e.g., Moqom)
- Social community borrowing marketplace – facilitating peer-to-peer connected borrowing and lending (e.g., Flender.ie)
- Crypto services from wallet to bank account – ensuring continuous crypto customer journey (e.g., TenX)
- Supply chain cryptocurrency-enabled cycle – disrupting all parts of the supply chain with crypto (e.g. PundiX)
- Inclusive finance – financial service-provision for underprivileged groups (e.g., M_Service through MoMo providing a range of services for the low-income family segment)
- Smart audit -improving data-enabled audit (e.g., CheckVentory)
- Handshake loan brokerage – providing a platform for lenders and borrowers to meet (e.g., Funding Societies matches SMEs with potential lenders)
- Research and market intelligence services – providing research-based insights (e.g., Smartkarma provides analytics and intelligence for investment sector)
- Evidence-based planning intelligence – facilitating planning through data science intelligence (e.g., Corlytics)
- Non-traditional insights through innovative analytics – using out-of-the-box analytics to generate/improve customer value (e.g., Eagle Alpha)
- Discounted or free money transfer services – efficient and cheap money transferring service provision (e.g., InstaRem, Elecpay)
All these secret sauce services are highly dependent on good-quality data as well as intelligence, which the data provides. Yet, with growing amounts of data available to FinTech analysts year by year, behavioural data science and behavioural AI provide new types of decision intelligence, which allows looking at data in creative, innovative, as well as responsible ways.
This, in turn, allows analysts not only to obtain new insights but also correct for noise and error in datasets as well as avoid the data overflow problems (especially those, when more data does not necessarily lead to better insights).
So what are the reasons for Behavioural AI to become one of the most promising tools in FinTech?
Looking Beyond Digital Marketing
Many of behavioural design specialists (and as a Behavioural Scientist I am also to some extent guilty as charged) tend to concentrate on descriptive and incremental insights which behavioural science can help generate.
Indeed, we know how to present/frame information to increase the level of understanding or aid decision-making; and we have become increasingly proficient in constructing these “nudges” and “framings” using, e.g., customer demographics.
However, behavioural AI allows us to do so much more.
As far as customer behaviour is concerned, we now have over a dozen of decision theories (both in a deterministic and stochastic form) which allow us to predict human decision making in risky and uncertain environments to an extent which can help both FinTechs as well as more traditional financial institutions to engineer products and services to fit the wants and needs of a specific consumer.
Moreover, these theories can now predict (i) when, where, and on what terms to offer these products and services to the customer to best fit their interests as well as (ii) how to do that in the most cost-effective way.
Hybrid modeling approaches between decision theory and machine learning, such as, for example, anthropomorphic learning, are now capable of better forecasting the future customer preferences such that FinTechs can match these preferences with suitable products.
Scalability and Mass-Customisation of Behavioural AI
Behavioural AI can not only tell us which financial products and services a particular individual might want tomorrow; how and when to present the information about these products and services to the customer; but also, because it combines behavioural with data science for practical applications, behavioural AI can tell us how to do that at scale.
We often talk about a continuous customer journey and how we can use a combination of behavioural science and technology to optimise a decision-making process.
Yet, not all customers are ready to accept this optimisation and much care should be exercised through the use of behavioural science models when a customer is approached.
For example, imagine that my bank invites me to a branch for a chat with a financial advisor.
This advisor tells me that their analytics team was looking at my data and judging from what I was shopping around for they think I might be interested in a savings account or an investment portfolio with a particular set of characteristics.
In this case, it is highly likely that this bank will have a happy customer (certainly, I will appreciate that they have done the research for me and will carefully study their recommendations).
However, if this bank tries the same trick with one of my colleagues, they are sure to lose a customer for good.
She would not be interested in staying with an organisation which she would describe as applying a “Big Brother” approach to customer service.
Note that in terms of demographic characteristics, I and the colleague in question are extremely similar. Under these circumstances, the only way to tell us apart would be to look at our decision-making patterns and construct a nice predictive behavioural model. Yet, this behavioural model would be useful only when it is combined with a machine learning algorithm in order to make sure that proposed solutions are scalable and “mass-customisable”.
Naturally, such mass-customisation options are much better placed at FinTechs rather than large banks, as FinTech firms are constantly looking for ways to innovate and update their personalisation strategies to capture larger market shares.
Forward-Looking Modelling for Behavioural AI
Behavioural AI allows predictive use of a product/service and customer characteristics to show what products and services a particular consumer would want to buy tomorrow. Therefore, Behavioural AI segments customers more efficiently and designs future services and products to fit the wants and needs of specific customer segments.
For example, using Behavioural AI we can analyse a large body of historical data combining house, mortgage, and customer characteristics (e.g., photos of properties, mortgage conditions, customer demographics and transactions data) to train a predictive algorithm which would match customers (according to their behavioural type) with the appropriate property (according to the property characteristics) and mortgage conditions.
The predictive model will be based on the Behavioural AI algorithm which will use a large number of property attributes as well behavioural attributes to minimise the decision time of the customer and increase the propensity for the customer to opt for an option, which would maximise their wellbeing.
Context Analytics and Demand Shaping
Contemporary personalisation techniques do not only require to make accurate predictions, but also to understand (i) what information should be provided to the customer to help their decision making; (ii) when to approach the customer; as well as (iii) shape the consumer demand to work with the future consumer needs on several levels.
Behavioural AI is capable of predicting (a) when, where, and on what terms to offer products and services to the customer to best fit their interests as well as (b) how to do that in the most cost-effective and least invasive way.
This approach also allows for “demand shaping” – i.e., predicting significant life events such as pregnancy, marriage, etc. and then matching customers in these predicted groups with customised products.
To give you a specific example, a few years back I had a child and was looking for a child savings account, while my bank was offering me credit card deals and loans. Naturally, had my bank used Behavioural AI to support their services, they would have been able to better respond to my needs.
Responsible Personalisation
Another challenge that needs to be addressed in the modern digital economy is the responsible delivery of personalised services.
One can write the best algorithm to make decisions about whether to offer or not to offer a particular mortgage deal to the customer.
However, the problem arises when one needs to justify to the customer why certain matches were made by the algorithm.
For example, if the algorithm offers different deals to different types of customers, it is necessary to ensure that this differentiation is done responsibly should a customer want to question or contest the FinTech’s decision outcome.
Behavioural AI tools allow us to incorporate values-by-design and human-right-by-design principles into our algorithm construction to address this problem.
Behavioural Digital Twins
A digital twin is, essentially, an exact digital copy of a physical entity, which could be living or non-living.
While digital twins have many pros and cons, their main advantage is that they take (computer) simulations to a new level; as they can be used to completely replicate conditions of a physical system and then, through the use of different scenarios test how these systems perform under stress or limited capacity.
Digital twins have recently become an established concept in data science and AI delivering and facilitating the adoption of disruptive technologies across many different sectors and domains including but not limited to FinTech, smart cities (see, e.g., the digital twin of Singapore), transportation, automotive, architecture, energy, farming.
Most recently digital twins made their entry into FinTech and are actively used in (cyber)security design as well as many other aspects of financial services. Behavioural AI provides an opportunity to design systems mimicking actual physical systems, dependent on human behaviour – i.e., behavioural digital twins and test outcomes using simulation methodology.
Interactive and Perceptional Design
I am one of those people who hates interacting with machines.
When calling a financial institution, I will press whatever button I need to press to get to a human operator. Yet, my sister, who is 7 years younger than me, is much more tolerant toward interactions with chatbots and automated systems.
Through Behavioural AI we can understand personal patterns with regard to automation interactions better and design better systems to increase customer satisfaction.
Behavioural AI would, for example, allow a FinTech company to customise my interactions and my sister’s interactions such that we both are satisfied with the service.
Naturally, it would direct me to a human operator and my sister to a bot.
Understanding Supply and Value Chains through Behaviour
Behavioural patterns propagate through entire supply chains and Behavioural AI can trace the journey of decisions, choices and behaviours in the chain as well as to recommend better ways of planning, managing, and controlling supply at different levels.
For example, by understanding substitutable and complimentary services within a particular business, Behavioural AI can better prepare a FinTech company to the demand shocked (e.g., those, which we have recently experienced due to COVID-19 crisis).
Behavioural AI for HR Systems
much behavioural AI thinking is going into the design of the new HR systems (not to be confused with the recent employee surveillance trends).
In contrast to surveillance economy measures, behavioural AI can inform how to create non-invasive, well-being-enhancing, productivity-increasing measures at the workplace as well as how to implement them at scale.
De-biasing decision making
in many cases, human decision making may be biased in different ways. People may exhibit conscious and unconscious cognitive as well as cultural biases, e.g., by discriminating against others for a variety of reasons.
Behavioural AI can spot these biases and suggest ways to build new decision support systems, which would highlight biases to decision-makers or even have built-in mechanisms for bias prevention.
Takeaways
Behavioural AI is a game changing technology, which has recently emerged from behavioural data science research.
It is currently impacting and will continue to influence the FinTech sector and financial services for the foreseeable future as it opens the door to new ways of understanding spending patterns, segmenting customers, designing wellbeing-increasing interventions, which benefits both consumers and companies. It is already clear that behavioural AI in the FinTech sector is a moneyball for money – the sector’s gift, which will keep on giving if used ethically and innovatively.